Relevance of the study
The relevance of the study lies in the fact that modern innovation projects face high risks and uncertainty regarding their successful realization and expected profitability. The use of artificial intelligence (AI) methods in economic and mathematical modeling makes it possible to assess these risks more accurately and to predict the profitability of projects with greater accuracy. This approach helps entrepreneurs and investors to make more informed decisions about investing capital in innovation projects, which, in turn, contributes to the development of the economy and stimulates innovation activity in society.
Purpose of the study
The purpose of this study is to assess the risks and predict the profitability of innovation projects using artificial intelligence methods within the framework of economic and mathematical modeling. The study aims to identify effective approaches to assessing the probability of successful realization of innovation projects, as well as to develop adequate models for predicting the potential profit from these projects.
Materials and methods of research
Both primary (statistical information) and secondary sources (scientific papers) were used for the study.
The research methods are: case study method, method of theoretical and practical analysis, method of comparative analysis.
Results of the study
In our time of rapid development of science and technology, science and technology are becoming more and more important and are used in many areas. In the financial industry, the use of technology has led to the emergence of a whole new artificial intelligence, and the integration of finance and technology has allowed people to discover new aspects of financial understanding. While the development of AI has brought many unforeseen risks to the financial industry, its innovative approach is also widely concerned and recognized. With the development of the financial industry and the integration of Internet technology, large amounts of information generated from financial transactions are effectively stored, effectively enabling the application of non-parametric statistical methods to control financial risks on the Internet. With the continuous improvement of social informatization, financial investors need to understand and process more financial information so that they can develop more intelligent investment strategies and carry out effective financial risk management.
In the information age, the rapidly developing big data technology, open and complementary global algorithms, Shannon's theory and Moore's law, and the nature of finding advantages and disadvantages have led to artificial intelligence technology, which has experienced two sharp ups and downs, to be reborn as a key element of digital ecology, not only creating a co-evolutionary ecological circle of the symbiosis of competition, cooperation, and dependence but also exposing a multitude of risks in information judgment. The financial industry is a key industry for the application of big data. Through big data analysis, it can provide early warning of possible online financial risks, so that relevant enterprise personnel can formulate reasonable preventive strategies in time and ensure the normal operation of online finance by analyzing multiple data. AI, as a product of the information age, provides people with great convenience. With the continuous development and widespread application of AI, the modernization of traditional industries, especially the finance industry, should be accelerated. As the most basic traditional industry, financial risk control should fully utilize AI to innovate and continuously improve the existing risk prevention and control system.
Risk assessment and profitability forecasting of innovation projects is a key element of the successful commercialization of STEM innovation.
Looking at the global experience, we see that innovation in STEM fields demonstrates the aspirations of academics, especially in the US, in creating and promoting scholarships for science and engineering students. The growth of scholarly work demonstrates the increasing interest of young professionals in research. The rapid development of STEM in the U.S. over the past decades has been greatly facilitated by both national policies and strong financial support from U.S. government agencies as well as private funding sources [2].
The rapid development of modern technologies and the constant emergence of innovative ideas require a comprehensive approach to analyzing potential risks and opportunities. Artificial intelligence is becoming an indispensable tool in economic and mathematical modeling, allowing a more accurate assessment of the probability of success of an innovative project and its expected profitability. The application of machine learning and data analysis methods makes it possible to take into account many factors that can affect the results of the project.
In this regard, the creation of an authoring toolkit for assessing the commercialization of STEM innovations becomes a necessity. The framework developed by experts in economics and finance takes into account the specifics of innovation projects and offers a comprehensive approach to their analysis [2].
This set of tools includes risk assessment methods, profitability forecasting, analyzing the competitive environment, and identifying potential strategies for project development. The use of the framework allows to provide more accurate and objective data for decision-making on further steps to commercialize innovations.
As for the use of a non-parametric statistical method based on the Internet platform, it can effectively combine traditional structural data analysis with unstructured data analysis to understand the overall operation state of the surveyed objects, and then generalize the existing rules and construct a change path for the operation state of enterprises. According to the constructed path, the risk points that need to be controlled in the financial behavior of the enterprise can be analyzed in real time. On this basis, we can evaluate the existing risk points point by point and finally realize financial risk control. Financial risk refers to any risk that may cause enterprises or institutions to lose assets, which is the uncertainty and volatility of enterprises' future earnings. The structure of the deep learning model of finance is shown in figure 1.
Fig. 1. Structure of building a deep learning model for financial risk assessment
Through measuring and understanding risks, appropriate measures and recycling schemes are taken to minimize risks and maximize profits. Internet finance is a manifestation of informatization, and the growth of financial activities on the Internet will lead to the emergence of corporate customers and related service information. Compared with traditional financial activities, collecting information on the Internet is more convenient, and even users' transaction records can be stored directly, which is convenient for building an Internet platform in the future and useful for communication between sellers and buyers. Faced with multiple data, how to fully and efficiently utilize it and avoid information asymmetry as much as possible is crucial for the financial industry. The effective application of AI in the financial industry can analyze and integrate this data, reduce financial risks to some extent, and effectively save labor costs.
With the development and popularization of information technology, most of today's financial transactions have moved from offline to online, which is why today's finance is also referred to as internet finance. The growth of the financial industry should be based on the huge number of commodity consumers, ensure people's financial security in online transactions, and seriously protect consumers' economic interests. At the same time, in order to comprehensively improve the effective growth of financial activities, the user data management should be well done, and the basic information of users will be directly collected into the enterprise information management system. Big data collects, classifies, stores and deeply analyzes structured and unstructured data from financial activities, and discover new knowledge, create new value and expand new opportunities [4, p. 12]. Figure 2 shows the process of networked precision marketing based on big data.
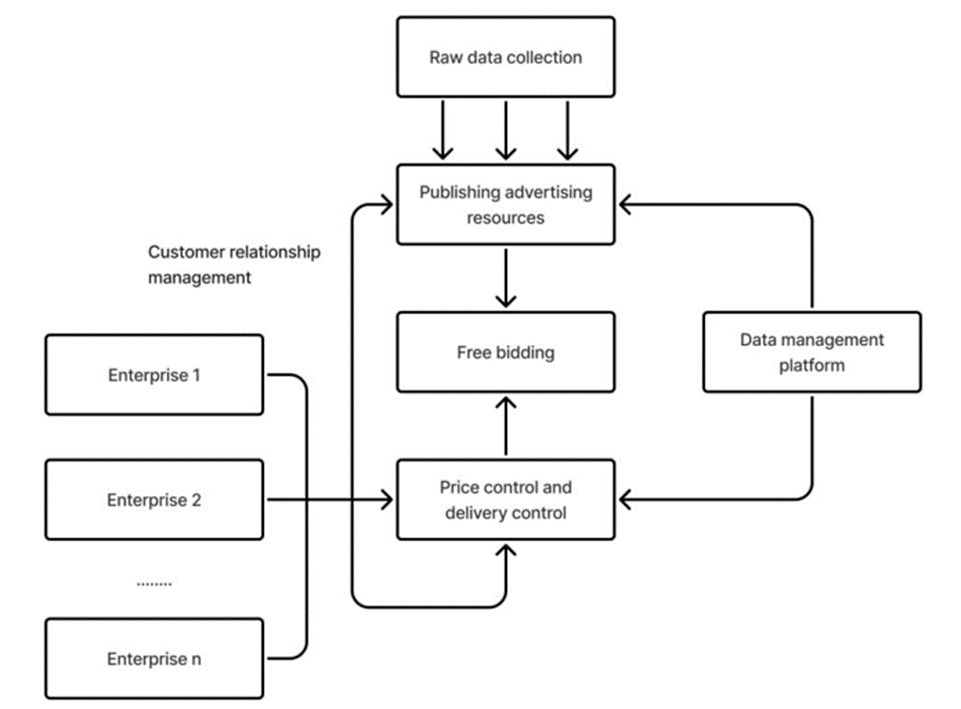
Fig. 2. Networked precision marketing based on big data
To further explore the value behind this data, big data mining technology has emerged, which requires our equipment to extract valuable information from complex data to realize its effective use.
Data is an indispensable source of information in the financial risk management process. Only by providing comprehensive information can we make effective financial risk decisions. Therefore, their collection is particularly important. It provides a vital information basis for financial risk management, guarantees the completeness of information data to a greater extent, and provides reliable assistance to improve the effectiveness of financial risk management decision-making. There are many factors affecting systemic financial risks, including asset maturity mismatch and irrational fluctuations in the financial system, macroeconomic imbalance and international economic factors. With the help of big data, comprehensive information can be obtained, and their mutual influence, interaction and propagation paths can be analyzed with mathematical statistics to provide scientific solutions to prevent systemic financial risks.
The risk early warning system is mainly designed to detect risks and alert the ongoing process of financial transactions over the Internet. The construction of this system should go through the whole process of financial activities to protect users' own interests. At the same time, it is necessary to reflect the decisions and documents made by the government and regulatory authorities in a timely manner to judge whether various transactional behaviors are in line with social norms.
At present, the foundation of information security is still weak, so we should actively encourage institutions engaged in the research and development of artificial intelligence technology to develop user information encryption technology. However, financial institutions generally lack the ability to research and develop user information encryption technology on their own. Therefore, financial institutions should strengthen cooperation with institutions that research and develop AI technologies in the process of applying AI in risk management, and utilize their technical advantages and professional research and development capabilities to prevent information security risks.
The outstanding advantage of AI is the integration of deep learning and big data. It can capture the sources of risk in the large amount of rapidly changing information in the financial market and provide scientific solutions for risk management. The AI-based financial risk identification system is shown in figure 3.
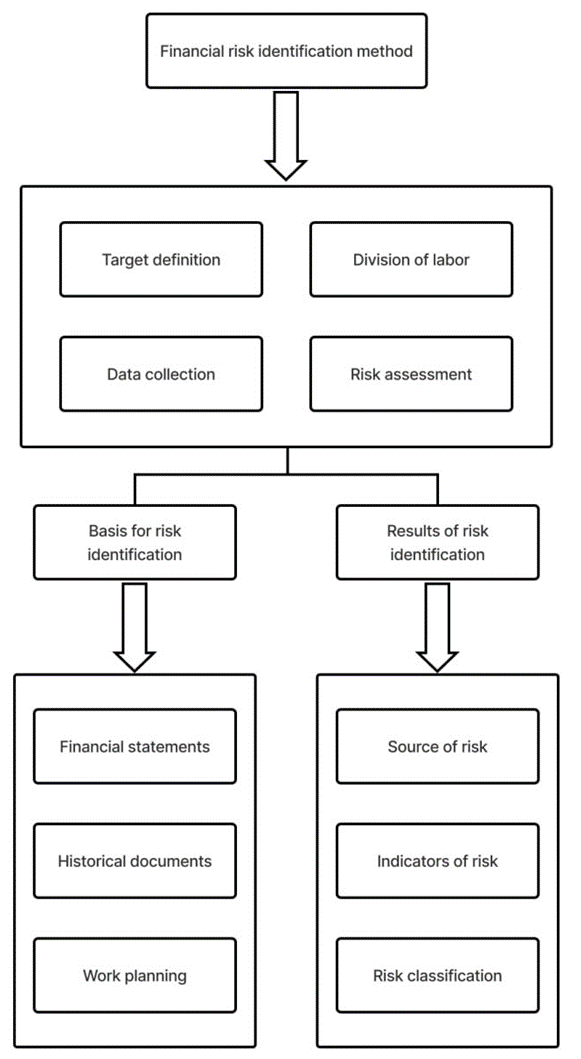
Fig. 3. Financial risk identification system based on artificial intelligence
In order to master the development characteristics and laws of financial risks, we should strengthen the application of artificial intelligence technology and isolate the core functions from the surface functions by using artificial intelligence technology, so as to provide the guarantee of improving the accuracy of financial risk management. The reasonable application of AI in financial risk management can accelerate data processing and analysis, and effectively save labor costs. Moreover, in order to fully implement the risk management system, we must first understand and correct the risk management process, so that each point of the process can be managed by the relevant department or individual to ensure the effective implementation of risk management rules and regulations. The comprehensive application of AI technology in financial risk management should also fully realize the advantages of AI technology in deep learning, big data fusion, etc. By utilizing this technical advantage of AI technology, we can accurately collect massive information about the financial market and risk factors, thus laying a more scientific, accurate, and comprehensive foundation for financial risk management decisions.
Conclusions
Risk assessment and profitability forecasting of innovative projects is a crucial step for the successful implementation of such projects. By using artificial intelligence techniques in economic and mathematical modeling, the accuracy and reliability of these estimates can be improved. Artificial intelligence allows analyzing large amounts of data and identifying hidden patterns and trends that can influence the success of an innovation project. Machine learning techniques can be used to create models that can predict the likelihood of a project's success and estimate its potential profitability.
Also, the use of artificial intelligence methods in economic and mathematical modeling, together with the author's set of tools for assessing the commercialization of STEM innovations, will maximize risk management and increase the chances of success in innovation. AI will allow to accumulate statistical information about various STEM innovations, perform calculations in a short period of time, and improve the accuracy of calculations and forecasts.
Moreover, artificial intelligence can help in identifying potential risks and threats to the project, which will allow developing a strategy to minimize them. Analyzing data using machine learning algorithms allows you to identify relationships between various factors and their impact on the project outcome.
Thus, artificial intelligence methods in economic and mathematical modeling can significantly improve the efficiency of risk assessment and forecasting the profitability of innovation projects, which will help prevent potential failures and ensure the successful implementation of the project.